The video tutorial on "Foundation Models and Fine-Tuning in AI" below delves into the key concepts of pre-trained foundation models and the fine-tuning process, and how they can be leveraged in developing advanced AI applications in finance and capital markets.
Click '>Play' above to learn more about Foundation models and fine-tuning
Foundation models are large pre-trained AI models, trained on massive amounts of data to generate high-quality language representations. They are designed to perform basic NLP tasks such as sentiment analysis, text classification, and machine translation, and are often used as a starting point for more advanced AI applications. These models save time and computational resources, as developers can avoid training models from scratch, and instead fine-tune them to their specific use case.
Fine-tuning is the process of modifying pre-trained AI models to meet specific use cases, where the user provides the model with a smaller, task-specific dataset. The model is then trained on this dataset to better perform the desired task, leading to greater customization and improved performance. Fine-tuning can be used to automate financial reporting, analyze investment bank research, financial news articles, and other primary sources, and quickly analyze changes in market sentiment relating to currency or interest rates and government bond yields.
One significant advantage of fine-tuning is that it allows developers to tailor pre-trained models like OpenAI's GPT-3 to specific business use cases. Fine-tuning is relatively quick and can be accomplished in a couple of hours, even for large-scale customizations, making it easy for organizations to develop their own bespoke version of a pre-trained AI model that has taken months to train.
The importance of fine-tuning is particularly evident in finance and capital markets, where the constantly changing market conditions require accurate, up-to-date information. For instance, asset managers can use sentiment analysis to rebalance their portfolios between risk-off defensive stocks and risk-on growth stocks, and commodities traders can leverage news articles to detect subtle shifts in supply and demand. Global macro hedge funds may use fine-tuned AI models to generate real-time signals that predict changes in currency or interest rates, and government bond yields.
In conclusion, the tutorial underscores the significant role of foundation models and fine-tuning in developing advanced AI applications. By providing a starting point for new AI applications and allowing for greater customization and improved performance, these concepts are transforming the AI industry, particularly in finance and capital markets.
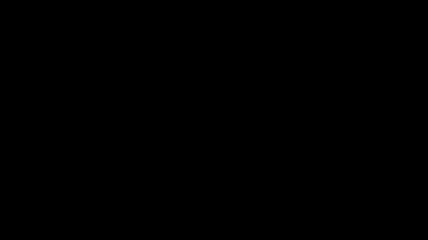